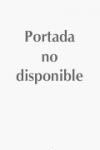
Machine Learning Applications in Industrial Solid Ash
Qi, Chongchong
Chen, Qiusong
Yilmaz, Erol
Machine Learning Applications in Industrial Solid Ash begins with fundamentals in solid ash, covering the status of solid ash generation and management. The book moves on to foundational knowledge on ML in solid ash management, which provides a brief introduction of ML for solid ash applications. The reference then goes on to discuss ML approaches currently used to address problems in solid ash management and recycling, including solid ash generation, clustering analysis, origin identification, reactivity prediction, leaching potential modelling and metal recovery evaluation, etc. Finally, potential future trends and challenges in the field are discussed. Offering the ability to process large or complex datasets, machine learning (ML) holds huge potential to reshape the whole status for solid ash management and recycling. This book is the first published book about ML in solid ash management and recycling. It highlights fundamental knowledge and recent advances in this topic, offering readers new insight into how these tools can be utilized to enhance their own work. Helps readers increase their existing knowledge on data mining and MLTeaches how to apply ML techniques that work best in solid ash management and recycling through providing illustrative examples and complex practice solutionsProvides an accessible introduction to the current state and future possibilities for ML in solid ash management and recycling INDICE: Part I : Industrial Solid Ashes 1. Background of industrial soild ashes 2. Current strategies for solid ash management and recycling Part II: Machine Learning Modelling 3. Historical background of ML 4. Introduction to ML techniques 5. ML modelling methodology Part III : Application of ML in solid ash management and recycling 6. Physiochemical properties of solid ash and clustering analysis 7. Accurate estimation of the solid ash generation 8. Evaluation of the trace elements pollution of coal fly ash using ML techniques 9. Metal recovery prediction using random forest 10. Rapid identification of amourphous phases in solid ash 11. Reactivity classification of solid ash using ML techniques 12. Forecast of uniaxial compressive strength of solid ash-based concrete Part IV : Future perspectives and challenges to adopting ML in solid ash management and recycling 13. Future perspective and opportunities in ML for solid ash management and recycling 14. Challenges to adopting ML in solid ash management and recycling
- ISBN: 978-0-443-15524-6
- Editorial: Elsevier
- Encuadernacion: Rústica
- Páginas: 314
- Fecha Publicación: 01/12/2023
- Nº Volúmenes: 1
- Idioma: Inglés