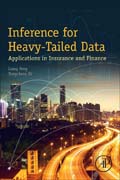
Inference for Heavy-Tailed Data Analysis: Applications in Insurance and Finance
Peng, Liang
Qi, Yongcheng
Inference for Heavy-Tailed Data Analysis: Applications in Insurance and Finance covers the heavy tailed data that frequently appears in social science, internet traffic, insurance and finance. Statistical inference has been studied for many years, including recent bias-reduction estimation for tail index and high quantiles with applications in risk management, empirical likelihood based interval estimation for tail index and high quantiles, hypothesis tests for heavy tails, and the choice of sample fraction in tail index and high quantile inference. These results for independent data, dependent data, linear time series and nonlinear time series are scattered in different statistics journals. This book puts these methods into a single place with a clear picture on learning and using these techniques. Contains comprehensive coverage of new techniques of heavy tailed data analysisProvides examples of heavy tailed data and its usesBrings together, in a single place, a clear picture on learning and using these techniques INDICE: 1. Independent Data: bias-corrected estimators, interval estimation, hypothesis tests, choice of sample fraction 2. Dependent Data: inference for mixing data, ARMA models, GARCH(1,1) models 3. Multivariate Regular Variation: Recent research on hidden regular variation, functional time series. 4. Applications: a tool-box in R will be applied to analyse data sets in insurance and finance
- ISBN: 978-0-12-804676-0
- Editorial: Academic Press
- Encuadernacion: Rústica
- Páginas: 200
- Fecha Publicación: 01/09/2017
- Nº Volúmenes: 1
- Idioma: Inglés