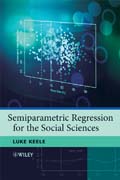
Semiparametric Estimation in the Social Sciences aims to provide an introduction, for both applied researchers and graduate students. It will be a rigorousbut not overly technical guide to using these techniques with social science data. Aimed at any social scientist that uses statistical models in his/her research, this book will examine all the key issues involved in the subject, andwill thoroughly explain the concepts underlying the statistical techniques, affording a full understanding to those with a minimal background in statistics. The potential of these methods is demonstrated through the inclusion of a variety of detailed empirical examples drawn from the social and political sciences. The readers knowledge will be further strengthened by the inclusion of exercises in each chapter. INDICE: Preface. 1. Introduction: Global vs Local Statistics. 1.1 The Consequences of Ignoring Nonlinearity. 1.2 Power Transformations. 1.3 Nonparametric and Semiparametric Techniques. 1.4 Outline Of The Text. 2. Smoothing and Local Regression. 2.1 Simple Smoothing. 2.1.1 Local Averaging. 2.1.2 Kernel Smoothing. 2.2 Local Polynomial Regression. 2.3 Nonparametric Modeling Choices. 2.3.1 The Span. 2.3.2 Polynomial Degree and Weight Function. 2.3.3 A Note On Interpretation. 2.4 Statistical Inference for Local Polynomial Regression. 2.5 Multiple Nonparametric Regression. 2.6 Conclusion. 2.7 Exercises. 3. Splines. 3.1Simple Regression Splines. 3.1.1 Basis Functions. 3.2 Other Spline Models andBases. 3.2.1 Quadratic and Cubic Spline Bases. 3.2.2 Natural Splines. 3.2.3 B-splines. 3.2.4 Knot Placement and Numbers. 3.2.5 Comparing Spline Models. 3.3Splines and Overfitting. 3.3.1 Smoothing Splines. 3.3.2 Splines as Mixed Models. 3.3.3 Final Notes on Smoothing Splines. 3.3.4 Thin plate splines. 3.4 Inference for Splines. 3.5 Comparisons And Conclusions. 3.6 Exercises. 4. Automated Smoothing Techniques. 4.1 Span By Cross-Validation. 4.2 Splines and Automated Smoothing. 4.2.1 Estimating Smoothing Through the Likelihood. 4.2.2 Smoothing Splines and Cross-Validation. 4.3 Automated Smoothing in Practice. 4.4 Automated Smoothing Caveats. 4.5 Exercises. 5. Additive and Semiparametric Regression Models. 5.1 Additive Models. 5.2 Semiparametric Regression Models. 5.3 Estimation. 5.3.1 Backfitting. 5.4 Inference. 5.5 Examples. 5.5.1 Congressional Voting. 5.5.2 Example: Feminist Attitudes. 5.6 Discussion. 5.7 Exercises. 6. Generalized Additive Models. 6.1 Generalized Linear Models. 6.2 Estimation of GAMS. 6.3 Statistical Inference. 6.4 Examples. 6.4.1 Logistic Regression: The Liberal Peace. 6.4.2 Ordered Logit: Domestic Violence. 6.4.3 Count Models: Supreme Court Overrides. 6.4.4 Survival Models: Race Riots. 6.5 Discussion. 6.6 Exercises. 7. Extensions of the Semiparametric Regression Model. 7.1 Mixed Models.7.2 Bayesian Smoothing. 7.3 Propensity Score Matching. 7.4 Conclusion. 8. Bootstrapping. 8.1 Classical Inference. 8.2 Bootstrapping - An Overview. 8.2.1 Bootstrapping. 8.2.2 An Example: Bootstrapping the Mean. 8.2.3 Bootstrapping Regression Models. 8.2.4 An Example: Presidential Elections. 8.3 Bootstrapping Nonparametric and Semiparametric Regression Models. 8.3.1 Bootstrapping Nonparametric Fits. 8.3.2 Bootstrapping Nonlinearity Tests. 8.4 Conclusion. 8.5 Exercises. Epilogue. Appendix: Software.
- ISBN: 978-0-470-31991-8
- Editorial: John Wiley & Sons
- Encuadernacion: Cartoné
- Páginas: 232
- Fecha Publicación: 08/02/2008
- Nº Volúmenes: 1
- Idioma: Inglés