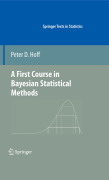
This book provides a compact self-contained introduction to the theory and application of Bayesian statistical methods. The book is accessible to readers with only a basic familiarity with probability, yet allows more advanced readers to quickly grasp the principles underlying Bayesian theory and methods. The examples and computer code allow the reader to understand and implement basic Bayesian data analyses using standard statistical models and to extend the standard models to specialized data analysis situations. The book begins with fundamental notions such as probability, exchangeability and Bayes' rule, and ends with modern topics such as variable selection in regression, generalized linear mixed effects models and semiparametric copula estimation. Numerous examples from the social, biological and physical sciences show how to implement these methodologies in practice. Provides a nice introduction to Bayesian statistics with sufficient grounding in the Bayesian framework without being distracted by more esoteric points The material is well-organized, weaving applications, background material and computation discussions throughout the book R examples also facilitate how the approaches work. A website with all of the data, Rfunctions and commands for all of the examples, homework solutions for instructors. INDICE: Introduction and examples.- Belief, probability and exchangeability.- One parameter models.- Monte Carlo approximation.- The normal model.- Posterior approximation with the Gibbs sampler.- The multivariate normal model.- Group comparisons and hierarchical modeling.- Linear regression.- Nonconjugate priors and the Metropolis-Hastings algorithm.- Linear and generalized linear mixed effects models.- Latent variable methods for ordinal data.
- ISBN: 978-0-387-92299-7
- Editorial: Springer
- Encuadernacion: Cartoné
- Páginas: 268
- Fecha Publicación: 01/08/2009
- Nº Volúmenes: 1
- Idioma: Inglés