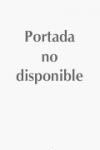
Modern data mining tools that predict future trends and behaviors for allowing businesses to make proactive and knowledge-driven decisions, demand fast andfully automatic clustering of very large datasets with minimal or no user intervention. In this volume, we formulate clustering as an optimization problem,where the best partitioning of a given dataset is achieved by minimizing/maximizing one (single-objective clustering) or more (multi-objective clustering) objective functions. Using several real world applications, we illustrate the performance of several metaheuristics, particularly the Differential Evolutionalgorithm when applied to both single and multi-objective clustering problems, where the number of clusters is not known beforehand and must be determined on the run. This volume comprises of 7 chapters including an introductory chapter giving the fundamental definitions and the last Chapter provides some important research challenges. Latest research on metaheuristic clustering INDICE: Metaheuristic Pattern Clustering – An Overview.- Differential Evolution Algorithm: Foundations and Perspectives.- Modeling and Analysis of the Population-Dynamics of Differential Evolution Algorithm.- Automatic Hard Clustering Using Improved Differential Evolution Algorithm.- Fuzzy Clustering in theKernel-Induced Feature Space Using Differential Evolution Algorithm.- Clustering Using Multi-objective Differential Evolution Algorithms.- Conclusions and Future Research.
- ISBN: 978-3-540-92172-1
- Editorial: Springer
- Encuadernacion: Cartoné
- Páginas: 300
- Fecha Publicación: 01/03/2009
- Nº Volúmenes: 1
- Idioma: Inglés